A Different Perspective
CAFNR researchers use UAV imagery and artificial intelligence to improve soybean breeding.
COLUMBIA, MO.
High yield potential is often the top trait crop breeders look at, as higher yielding crops can lead to more money in a producer’s pocket.
Researchers in the University of Missouri College of Agriculture, Food and Natural Resources (CAFNR) conduct a variety of trait-related trials through various breeding programs.
The Precision and Automated Agriculture Lab (PAAL) in the CAFNR Division of Food Systems and Bioengineering recently teamed up with researchers in the CAFNR Division of Plant Sciences to study yield estimation of soybean breeding lines under drought stress using unmanned aerial vehicle-based imagery and convolutional neural network. Their work resulted in a paper published by Biosystems Engineering.
“Breeding is a process to test plants with varieties of gene combinations and select the best combinations regarding their yield and other desired traits,” said Jing Zhou, a doctoral student in bioengineering and a member of PAAL who helped lead the project. “The more combinations get tested in the beginning, the higher the chance is to select the best lines with optimal gene combination. However, the capacity of breeding tests is limited by the required labor inputs to measure yield. It is almost impossible to harvest tens of thousands of progeny rows individually in a 9-acre field within a short harvest window.
“With the proposed method in this paper, human labor will be replaced by unmanned aerial vehicles (drones) and cameras. The 9-acre field, for example, can be harvested and measured by two people in just a few hours. Therefore, the increased breeding capacity of the soybean breeding program will result in the significant improvement of the breeding efficiency.”
Zhou said that in the published study, the group planted nearly 1,000 soybean breeding lines in three maturity groups under rain-fed conditions to test the drought tolerance. Aerial images were taken at three growth stages – late vegetation (V6), early reproductive (R1) and late reproductive (R6-R8). Seven image features associated with plant height, canopy color and canopy texture were selected to estimate the yield of each breeding line. A mixed convolutional neural network model was built to estimate soybean yield by taking the seven image features and two categorical features – maturity group and drought tolerance – as predictors.
A convolutional neural network is an algorithm, which can take in an inputted image, assign importance to aspects within the image and then differentiate those aspects from one photo to another.
“Projects in our lab focus on applying advanced technologies and methods to address agricultural challenges,” Zhou said. “In my doctoral training, I worked on characterizing key agronomic traits for soybean breeding with modern technologies in remote sensing, feature engineering and artificial intelligence (AI). Yield is the most important trait for soybean breeding. However, measuring soybean yield is labor-intensive and time-consuming, especially for progeny trials with tens of thousands of soybean plots planted in a season, which need to be harvest individually in a short time widow. This intensive labor investment in the breeding is one of the bottlenecks, limiting the breeding efficiency. Thus, we thought a more efficient tool should be developed to quickly and accurately measure soybean yield for breeders.”
Early results from the work showed that the image features collected at the early and late reproductive growth stages were comparably promising in estimating soybean yield. The predictive model could explain nearly 80 percent of the measured yield.
“Our tool can give the most accurate yield estimation in this research area and we are excited on this achievement,” Zhou said.
“Based on our observations and analysis over years, we are very confident about our models and image features. The results did prove that the technologies could replace the traditional yield measuring ways with neglectable errors. It means that work that took several men several weeks to complete can be reduced to a two-man job and be done in several hours.”
Jianfeng Zhou, assistant professor of agricultural systems technology in the Division of Food Systems and Bioengineering, is the principal investigator of the Precision and Automated Agriculture Lab and serves as Jing Zhou’s supervisor. Jianfeng Zhou was a collaborator on the project, as was Heng Ye, research scientist in the Division of Plant Sciences; Md Liakat Ali, post doctoral fellow in the Division of Plant Sciences; Pengyin Chen, who holds the David M. Haggard Endowed Professorship in Soybean Breeding at the Fisher Delta Research Center; and Henry Nguyen, professor in the Division of Plant Sciences.
“My supervisor, Dr. Jianfeng Zhou, has experience in predicting crop yield and encountered the challenges of poor model performance when he started it a couple of years ago,” Jing Zhou said. “But in recent years, AI is very popular in different industries and proved to be useful for agriculture problems. So we think it is worth trying to apply these complicated models to predict complicated yield.
“I did a literature review about this topic and realized that there is a blank. So we reached out to Dr. Pengyin Chen and Dr. Henry Nguyen who are very open-minded to modern technologies, and supportive. They provided soybean materials and research fields. Our group went to the field three times when soybeans were at different growth stages. We collected UAV images in multiple dimensions and some ground data to validate UAV measurements. I then processed the images and calculated a bunch of image features for each plot. I applied some feature engineering methods to select image features that were statistically related to yield. Finally I created a mixed-CNN model and tuned its parameters for the optimal results.”
The soybean lines that the group used in the project were planted at the Fisher Delta Research Center, located in Portageville, Mo. The center conducts a variety of soybean-related research, led by Chen.
“The Fisher Delta Research Center is located in a humid subtropical climate region with sandy soil that facilitates moisture stress development that is the target environment we need for the research,”
Zhou said. “The field has been used as an ideal location for drought research by University of Missouri scientists for several years.
Besides drought experiments, it is also ideal for flooding and herbicide studies due to its unique geographical advantages. They also have ongoing experiments for corn, cotton and rice. The technicians at Fisher Delta are experienced and conduct excellent field operations to help keep the experiments going.”
The research conducted at Fisher Delta and highlighted through the paper showcases one of CAFNR’s Grand Ideas in its strategic plan – Smart Farms. As the digital age comes to farms, technologies like robotics, AI, global positioning systems (GPS), sound-and-sight monitors, embedded sensors and big data analytics offer unprecedented opportunities to enhance efficiency and product quality. CAFNR is dedicated to studying these advances, as well as bringing them to farmers and producers across the state.
Zhou said that this research would provide a high-throughput yield estimation tool to soybean breeders and lead to more efficient variety development and release.
“Yield is a complicated trait that is controlled by a bunch of genes and conjunctively affected by genetic and environmental factors,” he said. “This research has proven that yield can be accurately estimated by an AI-assisted data analysis model and a group of image features taken over soybean canopies at 100 feet. This method can be applied to other aspects in breeding, such as detecting diseases, accessing drought/flooding stress injuries and estimating seed quality. The technologies help to enlarge breeding capacities, save resources and human labor, increase selection accuracy and eventually improve breeding efficiency. It has a potential to drive another ‘Green Revolution’ in agriculture production.” ∆
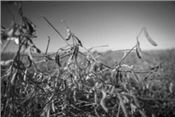
Crops with high yield potential are oftentimes the top trait breeders look at, as higher yielding crops can lead to more money in a producer’s pocket.
Researchers in the University of Missouri College of Agriculture, Food and Natural Resources (CAFNR) conduct a variety of trait-related trials through various breeding programs.
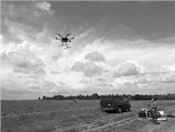
The Precision and Automated Agriculture Lab (PAAL) in the CAFNR Division of Food Systems and Bioengineering recently teamed up with researchers in the CAFNR Division of Plant Sciences to study yield estimation of soybean breeding lines under drought stress using unmanned aerial vehicle-based imagery and convolutional neural network. Their work resulted in a paper published by Biosystems Engineering.
Pictured is Jing Zhou flying a drone at the Fisher Delta Research Center.
Photo courtesy of Jing Zhou.